Finding Balance in the Age of Automation

By Martyn McDermott
6 min read
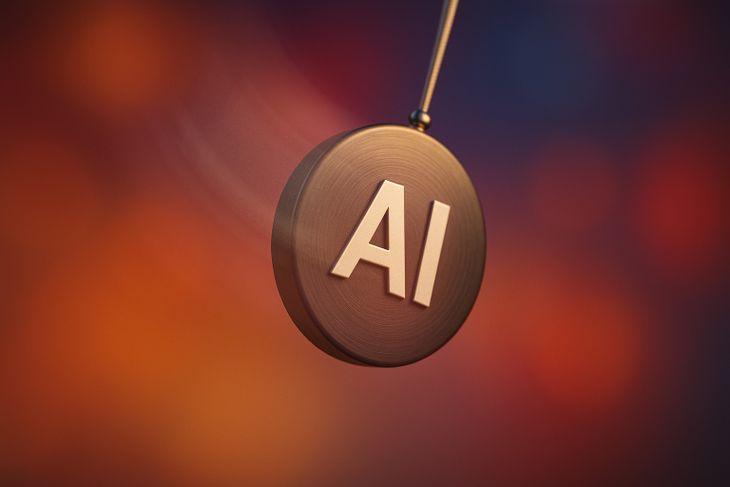
The AI Pendulum Swings
Klarna made headlines recently for walking back its AI ambitions. After months of touting automation as the future of customer service, the company is hiring human agents again. Turns out the bots were fast, cheap, and… not very good.
According to Klarna’s CEO, Sebastian Siemiatkowski, the AI chatbots delivered “lower quality” experiences. The kind that gets you decent metrics but angrier customers. Efficiency, meet your old friend: compromise.
I see this as a signal flare illuminating a larger, familiar, pattern in how technological revolutions unfold—one that might help us understand where we truly stand in AI's evolution.
The Great AI Overcorrection
Klarna's story follows a familiar script in other technological disruptions we've seen, in the simplest terms:
Hype rushes in: Companies eagerly implement new technology with promises of efficiency and cost reduction
Reality strikes: Implementation gets messy. Promises meet pushback.
Correction arrives: The pendulum swings back toward something more human.
We've seen this story before. Dot-coms. Mobile. Cloud. And now, AI. Except this time, the swings feel faster. Harder. Louder.
What makes this particularly interesting is that it's happening amid alarming statistics about AI implementation failures. In 2025, 42% of companies abandoned most of their AI efforts. That’s up from 17% the year before. Meanwhile, 70–85% of generative AI projects aren’t meeting ROI goals.
But strangely, budgets are still going up. Almost every large company is pouring more into AI—even though two-thirds admit they can’t get pilots past the starting line.
So where does that leave us?
Riding the Hype Curve
Gartner’s Hype Cycle has been around since the 90s. It charts how tech moves from buzz to burnout to something actually useful.
It goes like this:
Innovation Trigger: Initial breakthrough generates significant press and interest.
Peak of Inflated Expectations: A wave of publicity generates unrealistic enthusiasm and expectations. Everyone piles in. The future looks frictionless.
Trough of Disillusionment: Implementations fail to deliver on hype. Reality bites. Patience wears thin.
Slope of Enlightenment: The survivors learn what works. Their focused experimentation leads to true understanding of the technology's practical application.
Plateau of Productivity: Mainstream adoption begins as benefits become widely demonstrated and accepted. The noise dies down.
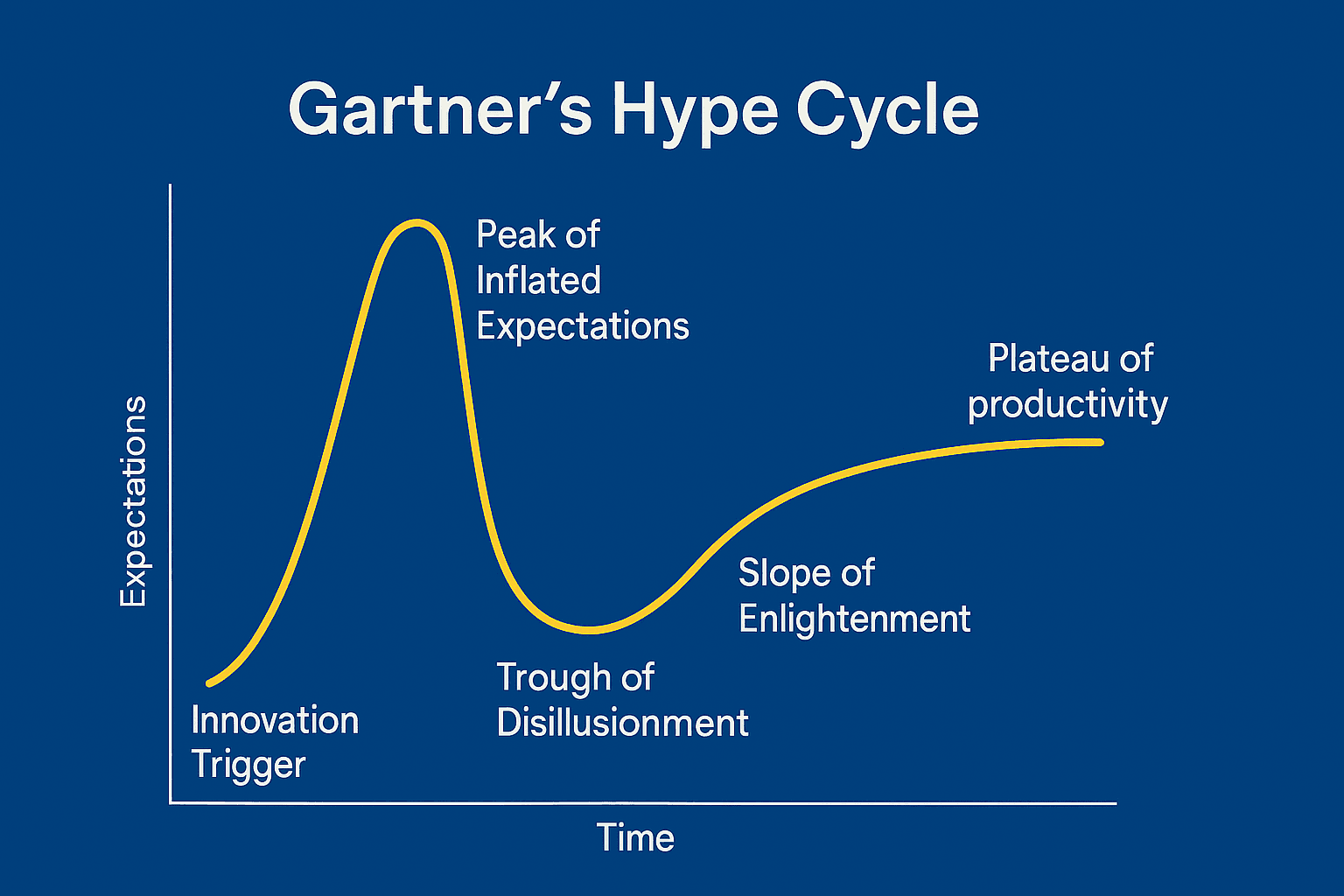
Now AI is on the ride.
Are We in the Trough Yet?
You could argue we’re entering it:
Only 47% of AI projects are currently profitable
High-profile reversals like Klarna (and Starbucks, quietly, last month)
The shiny promise of AI meets the awkward reality of “Sorry, I didn’t understand that”
Behind the scenes, data governance is a mess. Many firms are just not ready for scale.
But you could also argue we’re still near the top (Peak of expectations):
Investment keeps climbing
Niche applications are quietly succeeding
C-suite enthusiasm hasn’t cooled
New models keep getting better
The truth may be that different AI applications exist at different points on the curve simultaneously. Chatbots might be entering the trough while certain computer vision applications climb the slope of enlightenment.
It depends where you’re standing.
Inside the Klarna Reversal
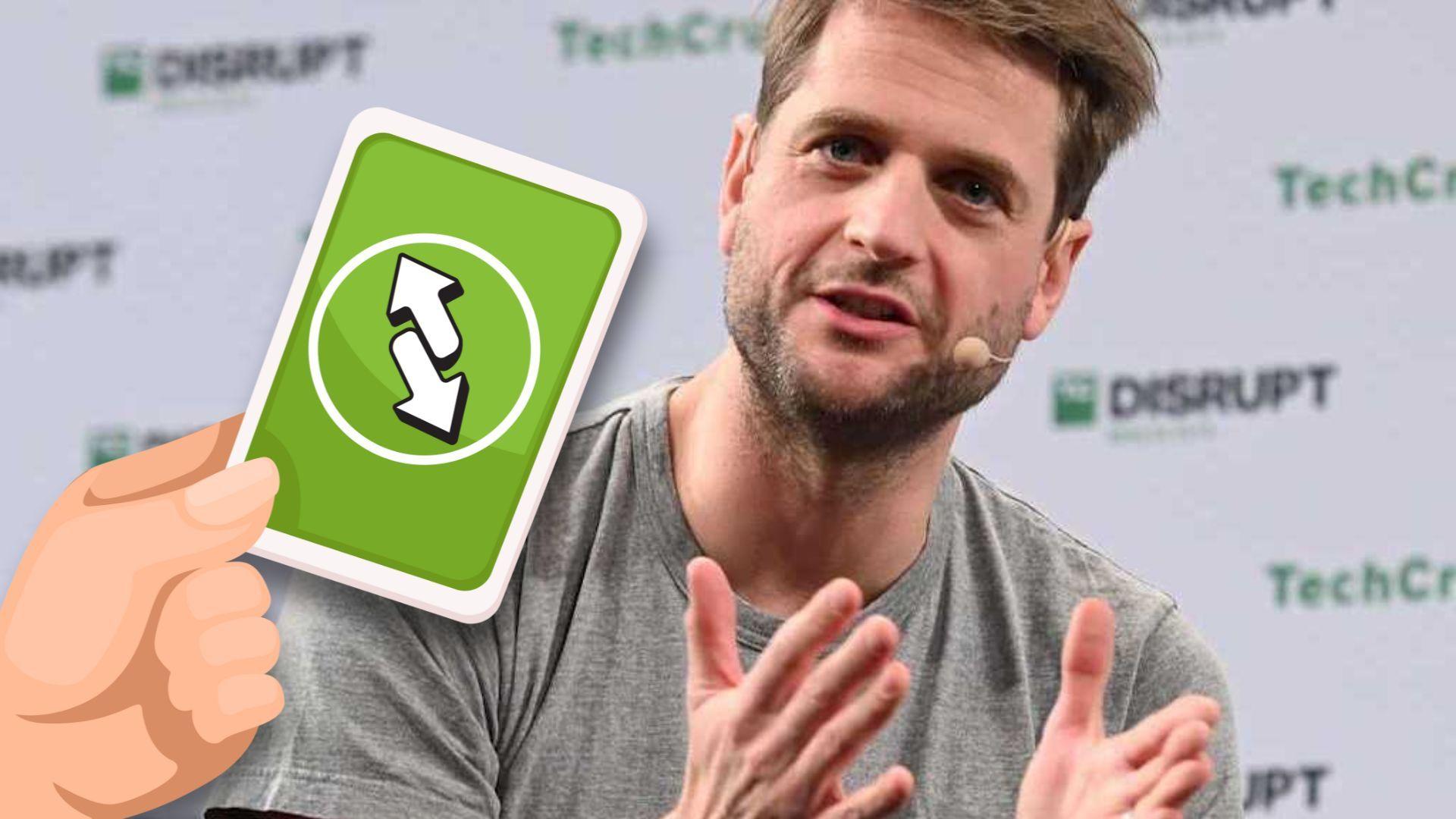
Diving deeper into Klarna's experience reveals fundamental challenges that many organisations face when implementing AI:
Klarna’s AI assistant made big claims: handling two-thirds of customer chats, solving issues in under two minutes.
But beneath the surface:
It struggled with nuance
Lacked empathy
Got stuck in loops
Couldn’t escalate properly
And worst of all, handovers broke the flow, forcing customers to start from scratch when a human finally stepped in
Fast and cheap. But brittle. Frustrating. Unkind.
These aren't just Klarna's problems, they represent the fundamental gap between current AI capabilities and the complex reality of human service interactions.
The False Binary: Beyond "AI vs. Humans"
Framing the conversation as "AI versus humans" creates a harmful false binary.
The real takeaway isn’t “AI failed.” It’s that we’re still designing systems like it’s either/or.
But the best outcomes don’t come from picking sides. They come from combining strengths.
Humans + AI > Either Alone
The organisations getting it right are doing things like:
Letting AI handle structure, humans handle sensitivity
Designing smart handoffs with continuity baked in
Creating feedback loops between teams and tools
Being honest with customers about who (or what) they’re talking to
It’s not replacement. It’s orchestration. Hybrid approaches embody a more mature understanding that the goal isn't to eliminate human involvement but to optimise the entire system for better outcomes.
Where Do We Go From Here?
If we are indeed approaching the trough of disillusionment for certain AI uses, that’s not a crisis. It’s a correction. Something natural and necessary that will ultimately lead to more sustainable implementations.
Signs to look out for that would confirm we're entering the trough include:
More public acknowledgments of AI implementation failures
Consolidation in the AI startup space
Increased focus on governance and practical implementation rather than capabilities
A shift from transformative rhetoric to incremental improvement language
The companies that will thrive:
Set grounded expectations
Lead with real-world problems, not shiny tech
Involve domain experts in the build
Design from day one for human-AI collaboration
Track meaningful outcomes, not vanity metrics
Embracing the Pendulum
This pendulum moment isn’t a sign that AI is broken, nor a win for the naysayers. It’s a sign that it’s growing up. Hype always fades. The trick is not to get caught in the swing. Or worse, try to build your whole business at the peak.
History shows that technologies with the greatest long-term impact often experience the most dramatic hype cycles. The internet didn't disappear after the dot-com crash, it became more deeply integrated into business fundamentals. Mobile computing didn't fade after early smartphone limitations, it evolved to transform how we interact with the digital world.
AI will follow a similar path.
The winners won’t be the ones who replaced the most people. They’ll be the ones who integrated thoughtfully. Who used the tech to raise the floor and the ceiling. Who knew when to automate—and when to care.
And the pendulum will continue to swing, but with each oscillation, we move closer to the balanced centre where technology serves human needs rather than merely replacing human functions. And that's progress worth waiting for.
What's your experience with AI implementation? Where do you think we are on the hype cycle? Share your thoughts in the comments below.
Written whilst wondering if my inbox is secretly managed by a Klarna chatbot.